“The more famous an expert is, the less accurate their forecasts are”
1 February 2016
It’s human nature to want to predict the future. Unfortunately, most of us are terrible forecasters. Including professional pundits. Yet some people have a way-above-average ability to forecast future events. Why? ing.world spoke with Dan Gardner, author, lecturer and, with Dr Philip Tetlock, co-author of the book Superforecasting: The Art and Science of Prediction.
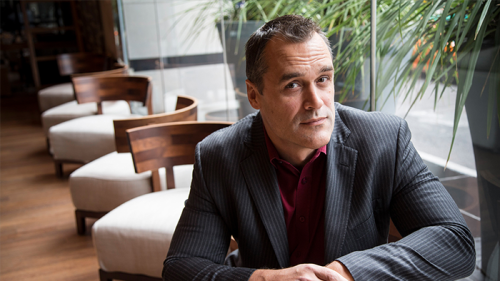
Could you explain the background to the book and the research it’s based on?
“The book came out of work by Dr Philip Tetlock, who is one of the world’s leading forecasting researchers. His first research programme was a real landmark amongst all sorts of folks with an interest in forecasting. Among them was the US intelligence community, which became interested in pursuing his idea that we could learn how to improve forecasting. Most organisations do a lot of forecasting, spend a lot of money on forecasting, and have little to no idea how good that forecasting is, or if they could make it better. This became the basis for further research, and the book.”
What do superforecasters have that makes them better forecasters than the rest of us?
“Phil's initial research looked at people whose job, to some degree, depended on making forecasts. So economists, political scientists, intelligence analysts, journalists. He had them make an enormous number of forecasts, and he found that the average forecast was about as a good as a chimpanzee throwing a dart, ie, random guessing. But this is a classic example of where the average obscures the reality, because there were two statistically distinguishable groups of experts. One did considerably worse than random guessing, but another did considerably better, which is to say that they had real foresight. Modest foresight, but real. The question is what distinguishes the two groups.”
And what does distinguish the superforecasters from the inferior forecasters?
“It wasn’t whether they had PhDs or access to classified information. What made a difference was the style of thinking, which Phil labelled foxes and hedgehogs after the ancient Greek poet, Archilochus, who said: ‘The fox knows many things, but the hedgehog knows one big thing’. For Phil, hedgehogs were those experts who had one big analytical idea that they thought allowed them to make good forecasts. They weren't interested in hearing other perspectives, other information or other ways of analysing problems. They wanted to keep it simple and elegant. They were much more likely to use words like “impossible”, or “certain”. Conversely, foxes didn't have one big analytical idea. They wanted to see other perspectives, hear other people’s views and learn about other ways of thinking. Of course, if you do that, your analysis is going to get complex, and messy, which is OK, because they're comfortable with complex and messy. But you're also probably going to give probabilistic forecasts – 65 percent, 70 percent – and be much less likely to use words like ‘impossible’ or ‘certain’.”
Can you explain a bit more about probabilistic thinking?
“If you accept that certainty is an illusion, you have to accept that everything is 1 percent to 99 percent, and it's a question of gradations between those. That's probabilistic thinking, and it's pretty much the opposite of what human beings do naturally. Humans naturally think in binary terms. ‘It will’ or ‘it won't.’ ‘It is or it isn't.’ If you force us to acknowledge uncertainty, we’ll say that something ‘may’ happen. ‘Maybe.’ But that's as fine-grained as our analysis gets. It’s what we call the three-setting mental model: yes, no, maybe. The probabilistic thinker drops yes and no in favour of fine degrees of maybe. You don't say that something is ‘likely’ to happen. You say that it is 65 percent probable, or 73 percent probable, or whatever. That level of clarity and precision is absolutely essential. Good forecasters are probabilistic thinkers and so very good at distinguishing fine degrees of maybe.”
So if the foxes are better forecasters, why do we only hear from the hedgehogs?
“The data shows an inverse correlation between fame and accuracy: the more famous an expert is, the less accurate their forecasts are. That happens for a very specific reason. Hedgehogs are the kind of people you see on television. The person who has one analytical idea, and who is very strong and confident in voicing his opinion – that person makes a great TV guest. A great public speaker. The person who says: ‘Well, I don’t have one big idea, but I have many ideas, and I’m thinking that there are multiple factors at work, some pointing in one direction, some pointing in the other direction…on one hand, on the other hand’ – that person makes a terrible TV guest.”
That’s a problem if you’re trying to improve forecasting…
“It’s a huge problem. The hedgehog expert gives you a nice, simple, clear, logical story that ends with “I’m sure”. That’s psychologically satisfying. That’s what we crave. The fox expert says: ‘Well, there are many factors involved, I’m not sure how we’re going to work out, balance of probabilities…’. That drives us crazy, because it isn’t psychologically satisfying. This is the deep paradox at work. The person who recognises that uncertainty cannot be eliminated is more likely to be an accurate forecaster than the person who is dead sure they’ve got it all figured out.”
Are there things that are simply too big to forecast? For example, the impact of Brexit?
“Usually with questions like this, we have people, usually in the media, taking contrary positions. They marshal their arguments and shout at each other. ‘Brexit will be a disaster.’ ‘Brexit will go swimmingly.’ We advocate breaking big issues into finer-grained questions like, say: “If Brexit negotiations begin by this date, will the pound be above or below this level by the following date?” That one question doesn’t settle it, obviously, but if you ask dozens of fine-grained questions like that, you can aggregate the answers and test whether Brexit works out well or works out poorly.”
Of course, that only tells you who was right in retrospect, not who will be right going forward.
“That’s true. But if you face a similar circumstance, and if you have somebody who has demonstrated, with evidence, that they really understood the dynamics that were at work last time, that’s probably somebody you should be listening to the next time there’s an analogous situation. Conversely, if you have somebody who clearly didn’t have a clue what was happening, that’s probably somebody you shouldn’t be getting your forecasts from. That sounds blindingly obvious, and yet you would be amazed at how many sophisticated individuals judge the quality of a forecaster based on their title, how many books they sell, how famous they are, how many times they’ve been to Davos. They do not judge them on their scientifically tested forecasting record.”
Which is why you advocate testing and measuring forecasters?
“It’s essential because, if you’re a consumer of forecasting, you want to know that you’re getting quality forecasting. There’s only one way to know that, isn’t there? Yet, amazingly, consumers of forecasting spend enormous amounts of money on forecasting whose quality they do not know. It may be wonderful forecasting. It may be dreadful forecasting. They simply do not know. The other reason why testing is essential is for the forecaster’s own good. If you never get feedback, or you only get fuzzy feedback, you can never improve.”
Can the rest of us learn to be superforecasters? Can people who are poor forecasters learn to be good forecasters?
“Absolutely. For this reason: you may be naturally inclined to engage in very fox-like analysis and think in probabilistic terms, but if you don’t actually do that, you won’t get the results in your forecast. Conversely, even if you are not naturally inclined to think like a fox, and in probabilistic terms, if you make yourself do it because you know it works, you will get the results. It’s a question of what you do, not what you are.”
Any tips for people who want to become better forecasters?
“I would say stay humble, and practice. Intellectually humble: appreciate that nothing is certain, that you have to think probabilistically, that you have to think about your own thinking to find those mistakes, and, most importantly, you have to practice. Forecasting is a skill like any other. You have to get out to the practice range and do it over and over again, and you have to get clear, accurate and timely feedback.”
Big Data, algorithms and machine learning seem to be proposed as the answer to every problem. Could they also help us to forecast better?
“There’s a lot of really interesting stuff being done with big data, algorithms and machine learning in forecasting, but the more interesting question is what happens to the humans who are forecasting? What happens when the machines meet the humans? Will the machines put the humans out of work? A lot of people say yes, and point to Deep Blue beating Garry Kasparov. But if you look at what’s happening in the chess world, there are now competitions in which people can choose to be human decision-makers, to play the game entirely using a chess program, or to combine a chess program with the human making the ultimate decisions. Guess what happens? Machine plus human beats both machine alone and human alone. And a lot of people think this will also be the case in other fields as well. The idea of a wise man forecasting the future will become ridiculous, but that doesn’t mean that human beings will be out of the forecasting business. It will be a wise person with a machine. They will work together, and they will produce better forecasts than either could separately.”
Is there a limit on how far ahead we can realistically expect to forecast?
“This is one of the great questions that needs to be seriously pursued. The gold standard is meteorology. Weather forecasts up to 24 hours ahead are very accurate. Forty-eight hours and 72 hours out they become progressively less accurate, but still quite good. You get to six or seven days out and you’re getting close to random guessing. A two‑week forecast? It’s useless. We know this because meteorologists make lots of forecasts that can be analysed. As a result, meteorologists have a very clear idea of their forecasting horizon. The problem is that in so many other fields, we don’t know what the forecasting horizon is, and, chances are, it’s going to vary widely from field‑to‑field. For example, the price of gold will have a different forecasting horizon to the price of oil. What is the forecasting horizon? We really, really don’t know, and the only way that we will know is if we start making numeric forecasts in large numbers, and then analysing the data.”
Earlier, you mentioned organisations not knowing whether their forecasts and forecasters can be relied on. You advise organisations, including the Canadian prime minister’s office, on how to make better decisions. What do you tell them?
“It’s exactly the same principle. First, if accuracy is the goal, you have to ask if you have created, or can create, an environment in which you reward accuracy, and only accuracy, because that’s essential. So often there are other goals in forecasting. You want the forecast to impress the client, to surprise people, to attract attention, to please your boss. All these things detract from accuracy, and one of the reasons why superforecasters are so good is that have only one goal: accuracy. Second, your organisation’s forecasters need clear, accurate and timely feedback on the accuracy of their forecasts. If they are not getting that then you have to create systems that will ensure they do. It’s the only way to improve.”
Final question. If you could be a banker for a day, what would you do regarding forecasting?
“A bank is in a wonderful position to improve forecasting, because you are awash in numbers and making forecasts all the time. So I would advise any banker to create an environment where they’re getting feedback about forecasts to their forecasters. You should be looking at setting up systems that give you that clarity of feedback. Phil uses the term "forecasting tournaments", and if I were in charge of a very large bank, I would have the equivalent of an internal forecasting tournament running all the time. Literally all the time. Just constantly churning out data, which individuals could use to judge and improve their own performance, and managers could use to judge and improve the bank’s overall performance.”
This interview was published before in ing.world magazine (2016).